In the rapidly evolving field of human-robot interaction (HRI), the integration of Artificial Intelligence (AI) into mechatronic systems represents a paradigm shift towards creating more intuitive, safe, and effective interactions. This integration, crucial for the advancement of Industry 4.0 and beyond, demands rigorous evaluation methodologies, performance metrics, and the development of personalized models that cater to diverse user needs and contexts.
The Importance of Rigorous Evaluation
For AI-enhanced mechatronic HRI to truly augment human capabilities without causing disruption, it is essential to adopt robust evaluation protocols that extend beyond mere demonstrations of functionality. These evaluations must affirm that AI models respond safely and effectively in real-world scenarios, highlighting the necessity for standardized protocols, metrics, and benchmarks. Such rigor ensures that advancements are meaningfully assessed, contributing to a shared understanding of progress within the research community.
Performance Metrics and Benchmarks
Evaluating the effectiveness of AI integration into mechatronic systems requires a balance between quantitative system metrics and qualitative human feedback. Performance indicators such as safety, explainability, reliability, usability, comfort, and trust play pivotal roles in determining the success of these integrations. Furthermore, benchmarks established through competitions and standardized challenges facilitate a comprehensive capability assessment, pushing the envelope of what is achievable through incremental innovation.
Enhanced Perception and Decision-Making
The ability of robots to accurately perceive and interpret human states, intentions, and commands is foundational for effective HRI. Advances in deep neural networks (DNNs) have significantly improved robots’ perceptual capabilities, enabling them to respond more accurately and sensitively to human requests and situational contexts. However, the journey doesn’t stop at perception; intelligent decision-making algorithms are essential for planning appropriate responses to perceived information, ensuring robots can execute tasks harmoniously alongside humans.
Bio-Inspired Control and Natural Interaction
Adopting bio-inspired sensorimotor controls facilitates more natural and safe physical interactions between robots and humans. These controls, learned through interaction experiences, enable robots to adapt their movements for cooperative tasks intuitively. Moreover, fostering natural interaction channels—encompassing audio, visual, and physiological signals—enhances communication, making HRI more intuitive and effective.
Personalization: The Key to Effective Long-Term HRI
General AI models offer broad accessibility, but adapting behaviors to individual human profiles leads to more meaningful interactions. Personalized models, which evolve based on interaction histories and contextual understanding, promise enhanced engagement, task efficiency, and assistance tailored to personal preferences and needs.
Addressing Open Issues and Future Outlook
While significant strides have been made in integrating AI into HRI, challenges remain, including ensuring safety in extreme scenarios, overcoming sample complexity constraints, and improving model explainability. Ongoing research aims to refine learning from demonstration techniques, develop hybrid architectures, and enhance transparency to maintain user trust in increasingly autonomous systems.
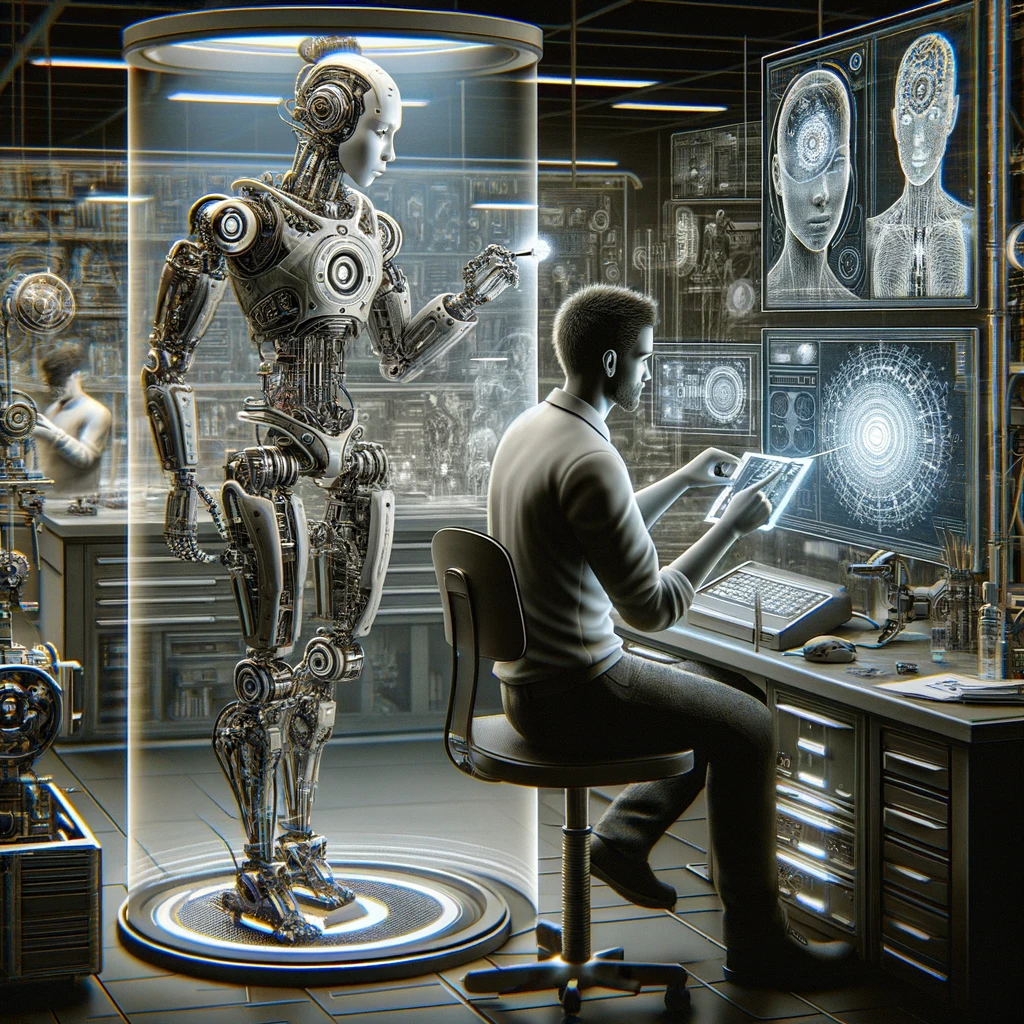