Introduction
The Rising Importance of AI Inference in Business
In the fast-paced digital era, artificial intelligence (AI) has transitioned from a futuristic vision to a core component of modern business strategies. Among its myriad applications, AI inference emerges as a pivotal element, especially within the scope of deep learning technologies. This article navigates through the intricacies of AI inference, delineating its role in enhancing competitive edge, streamlining operations, and fostering innovation across various sectors.
Understanding AI Inference
Defining AI Inference: From Basics to Business Impact
AI inference refers to the process of using trained AI models to make predictions or decisions based on new data. It’s the practical application of AI, where the ‘thinking’ happens, and insights are drawn. This process is crucial for businesses looking to deploy AI in real-world scenarios, from enhancing customer experiences to optimizing operational efficiencies.
Classical Machine Learning vs Deep Learning: A Comparative Analysis
Distinguishing between classical machine learning and deep learning is vital for understanding the scope of AI inference. Classical machine learning, with its simpler algorithms and fewer parameters, offers ease of interpretation and deployment. In contrast, deep learning, characterized by its complex neural networks, excels in handling vast amounts of data, albeit with higher computational requirements. This section delves into the pros and cons of each, aiding businesses in making informed decisions about their AI strategies.
The Computational Backbone of AI Inference
Navigating the Computational Demands of Deep Learning
The shift towards deep learning models brings to light significant computational challenges. These models require robust infrastructure to process the complex algorithms and massive data sets effectively. This part explores the computational demands of deep learning and the advancements in hardware and software that make these technologies accessible to businesses.
Hardware and Software Solutions for AI Inference
Specialized hardware, such as GPUs, and optimized software frameworks are critical in overcoming the computational hurdles of AI inference. This section reviews the latest developments in this area, offering insights into how businesses can leverage these solutions to enhance their AI capabilities.
Inference Patterns and Their Business Implications
Exploring Real-time, Batch, and Streaming Inference
AI inference can be categorized into three primary patterns: real-time, batch, and streaming. Each pattern serves different business needs, from instant decision-making to processing large volumes of data efficiently. Understanding these patterns is crucial for designing AI systems that align with specific business objectives.
Choosing the Right Inference Pattern for Your Business
Selecting the appropriate inference pattern is a strategic decision that can significantly impact the effectiveness and efficiency of AI applications. This section provides guidance on evaluating business needs and choosing the most suitable pattern for optimal results.
Architectural Blueprint for AI Inference Systems
Key Components and Design Considerations
Designing an AI inference system involves intricate planning and a deep understanding of both the technical and business aspects. This part outlines the essential components of an AI system, including model management, data processing, and infrastructure requirements, providing a roadmap for building effective and scalable AI solutions.
Optimizing AI Systems for Scalability and Efficiency
Scalability and efficiency are paramount for the sustainability of AI systems. This section offers best practices for optimizing AI inference architectures, ensuring they can handle growing data volumes and complex computations without compromising performance.
Overcoming Challenges in AI Inference Deployment
Best Practices for AI Model Management and Optimization
Deploying AI inference systems entails various challenges, from managing model lifecycle to optimizing computational resources. This part discusses strategies for overcoming these obstacles, emphasizing model management, performance tuning, and cost-efficiency.
Frameworks and Tools: Making the Right Choice
The selection of frameworks and tools plays a crucial role in the development and deployment of AI systems. This section reviews the leading options available to businesses, highlighting their features, advantages, and considerations for making an informed choice.
Future Trends in AI Inference and Business Applications
Emerging Technologies and Their Potential Impact
As AI continues to evolve, keeping abreast of emerging technologies and trends is essential for businesses aiming to stay ahead of the curve. This part explores future directions in AI inference, including advancements in algorithms, hardware, and applications, offering insights into how these developments could reshape industries.
Preparing for the Future: Strategic Insights for Businesses
Adapting to the rapid advancements in AI requires strategic foresight and planning. This section provides actionable advice for businesses looking to leverage AI inference for long-term success, emphasizing the importance of continuous learning, investment in technology, and fostering a culture of innovation.
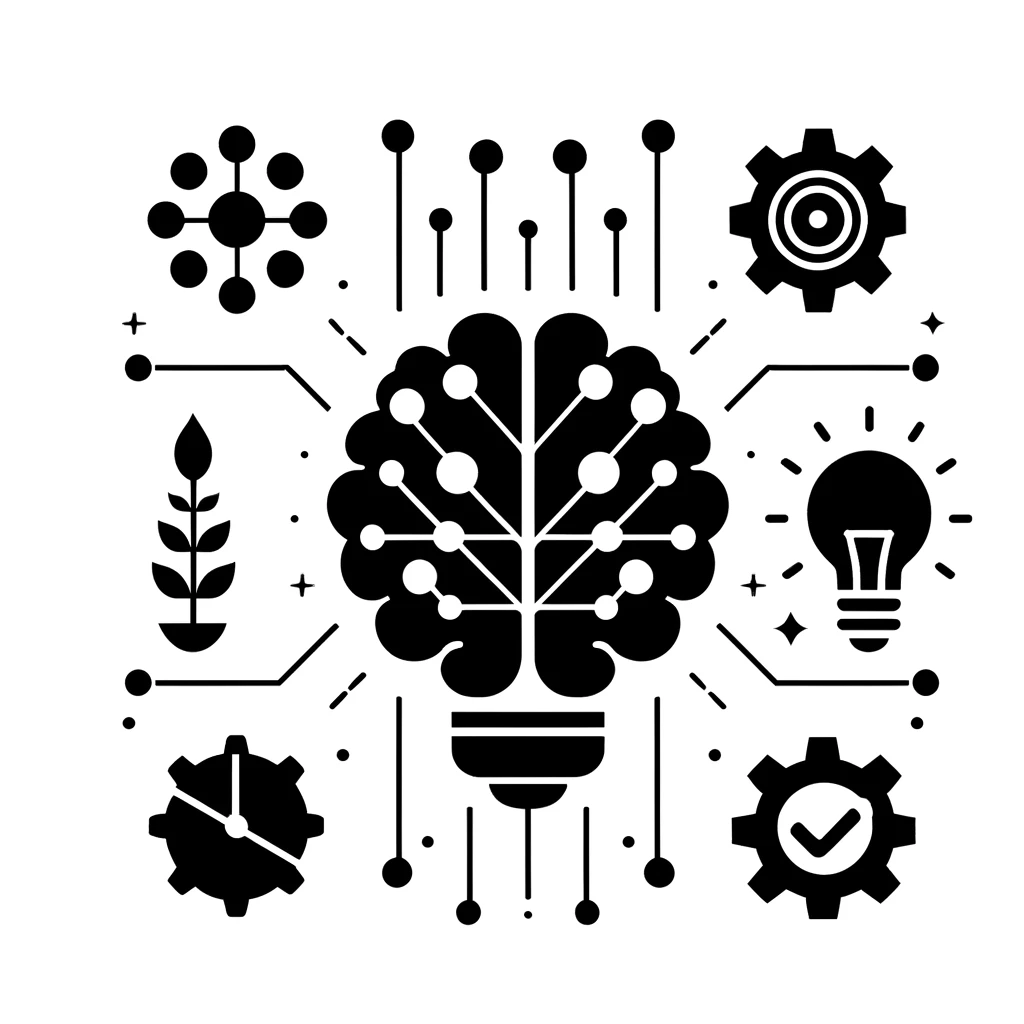