The integration of Large AI Models (LAMs) into bioinformatics and medical diagnosis is reshaping our approach to understanding biological systems and improving patient outcomes. AlphaFold2, a seminal development by DeepMind, represents a paradigm shift in protein structure prediction. Utilizing the Transformer architecture, originally designed for natural language processing, AlphaFold2 employs an innovative attention mechanism that models the spatial relationships between amino acid residues, enabling the prediction of protein folding with unprecedented accuracy. This breakthrough not only accelerates drug discovery but also opens new avenues in personalized medicine by elucidating the molecular basis of diseases.
AI-driven models like ChatCAD and HeartBEiT are pioneering the application of deep learning and natural language processing to enhance diagnostic precision. ChatCAD leverages LAMs such as ChatGPT to analyze and interpret medical images, integrating findings with patient data for comprehensive diagnoses. HeartBEiT, on the other hand, uses a foundation model trained on extensive electrocardiogram datasets to predict cardiac conditions, showcasing the potential of AI in predictive healthcare.
These developments underscore the crucial role of machine learning algorithms and deep learning frameworks in processing and analyzing vast datasets, paving the way for more accurate and personalized medical interventions.
Advancements in Medical Imaging and Informatics Through Deep Learning
The application of LAMs in medical imaging and informatics has led to significant advancements in the analysis, interpretation, and management of medical data. Models such as SAM (Segment Anything Model) have demonstrated remarkable flexibility across various imaging modalities, employing deep learning techniques to perform zero-shot segmentation tasks. This adaptability is crucial in medical imaging, where diverse data types and formats prevail.
Med3D further exemplifies the potential of deep learning in processing 3D medical images, utilizing convolutional neural networks (CNNs) to analyze volumetric data across different medical domains. This model’s ability to leverage multi-domain datasets highlights the evolving nature of AI in capturing the complexity of human anatomy and pathology.
In medical informatics, GatorTron and BioBERT represent significant advancements in natural language processing within the healthcare sector. GatorTron’s success in clinical documentation and BioBERT’s impact on biomedical research demonstrate how transformer-based models can efficiently handle domain-specific language, facilitating a deeper understanding of patient records and scientific literature.
These technical innovations reflect the ongoing evolution of AI methodologies, including CNNs, recurrent neural networks (RNNs), and attention mechanisms, in enhancing healthcare services and research.
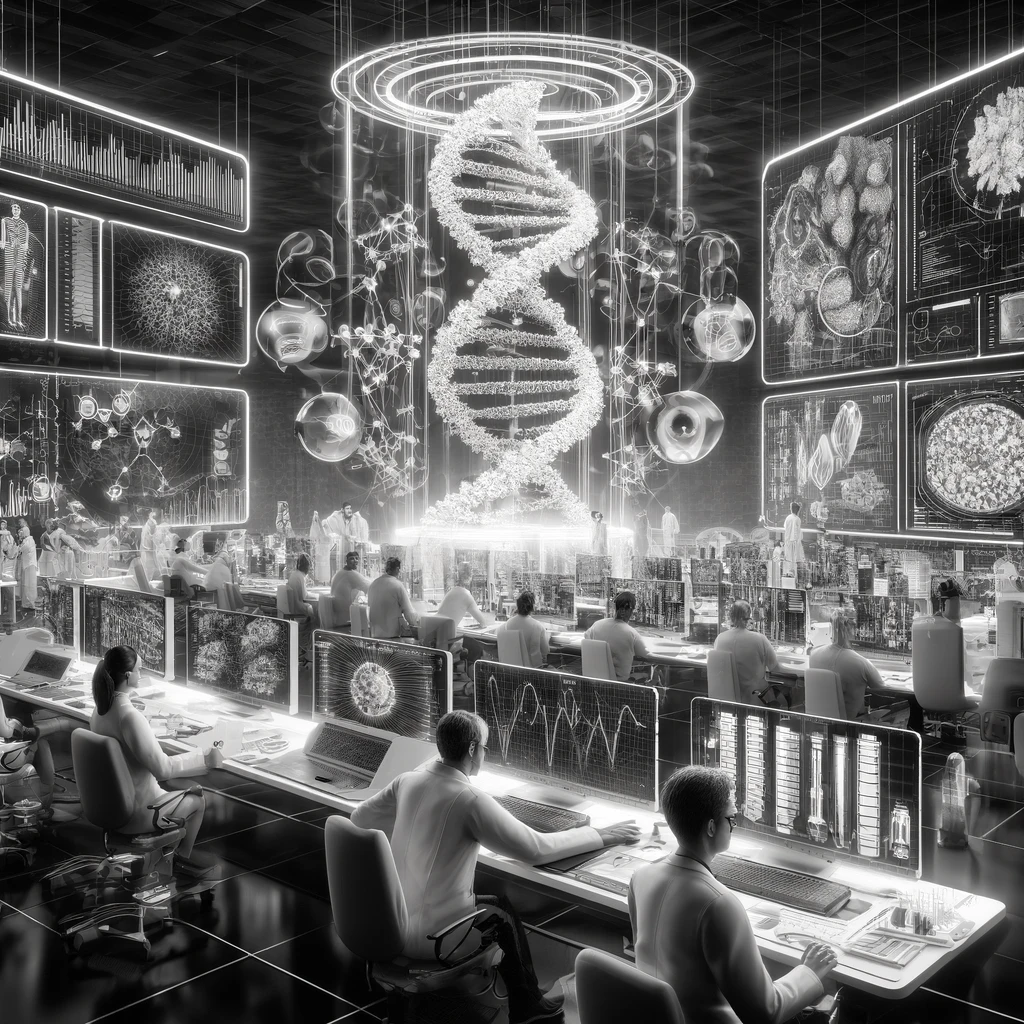