The application of Artificial Intelligence (AI) and Machine Learning (ML) to predictive maintenance represents a significant leap forward in ensuring the reliability and efficiency of energy production systems. This approach, known for its capability to analyze vast datasets to forecast potential equipment failures before they materialize, has been instrumental in minimizing downtime and bolstering the operational efficiency of energy infrastructures, particularly wind turbines. This article explores the latest advancements, successful applications, and the sophisticated algorithms driving this transformative technology.
Unveiling the Power of Predictive Maintenance in Energy Systems
Predictive maintenance harnesses AI and ML to sift through real-time and historical data, identifying patterns and anomalies that precede equipment malfunctions. This data-driven insight allows maintenance teams to act preemptively, averting failures that could disrupt energy production and supply. The heart of its success lies in the ability to accurately predict the lifespan of components and machinery, scheduling maintenance activities at the most opportune times to ensure maximum operational efficiency and longevity.
Latest Advancements and Applications
Recent advancements in predictive maintenance have been particularly impactful in the renewable energy sector, with wind turbines emerging as a prime beneficiary. The adoption of AI-driven predictive maintenance in wind energy has led to significant improvements in turbine reliability and efficiency. For instance, AI models can now predict gear failures or blade degradation by analyzing vibration patterns, sound frequencies, and other operational parameters. This proactive approach not only extends the operational life of the turbines but also enhances energy output consistency.
One of the landmark applications of predictive maintenance has been observed in offshore wind farms, where the cost and logistical challenges of maintenance are considerably higher than their onshore counterparts. AI-driven systems have been successfully deployed to monitor turbine health remotely, predict failures, and schedule maintenance with minimal human intervention, thus reducing the risk of unexpected downtimes and optimizing energy production.
The Algorithms at the Forefront
The efficiency of predictive maintenance in energy systems is largely attributed to a variety of sophisticated algorithms that underpin AI and ML models. Among these, the following are particularly noteworthy:
Time Series Analysis: Utilized for analyzing sequential data to forecast future states of machinery based on historical patterns. Algorithms such as ARIMA (AutoRegressive Integrated Moving Average) and LSTM (Long Short-Term Memory) networks are widely employed for this purpose.
Anomaly Detection: This involves identifying outliers in operational data that might indicate impending equipment failures. Techniques like Isolation Forests and One-Class SVM (Support Vector Machine) are commonly used for their high accuracy in anomaly detection.
Regression Analysis: Essential for modeling and predicting the expected lifespan of components. Linear regression and its more complex counterparts like polynomial regression have shown great promise in predicting the time-to-failure of critical parts.
Reinforcement Learning: Although still in its nascent stages of application in predictive maintenance, reinforcement learning shows potential in optimizing maintenance schedules by learning the best actions to take based on the current state of the system to maximize uptime and energy production.
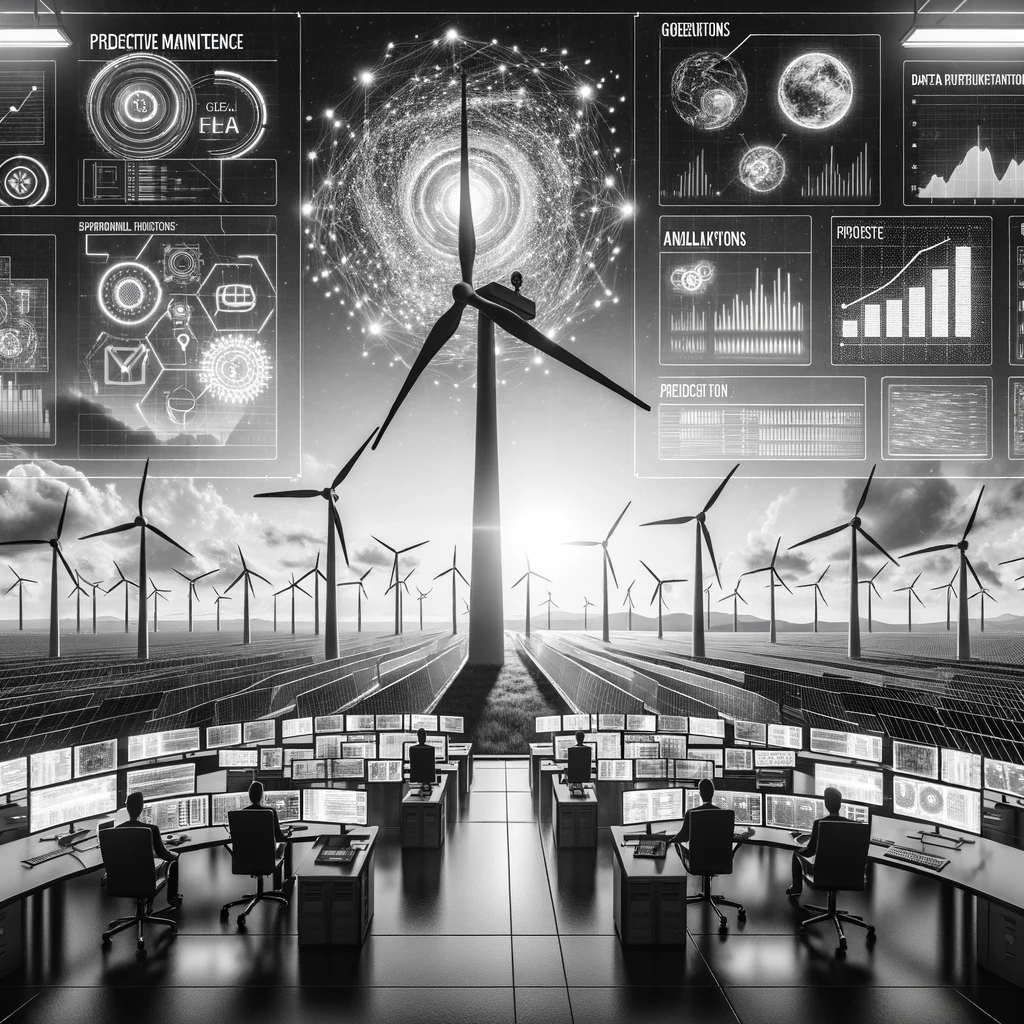